Examining Copyright Challenges in Training AI Models on Massive Datasets
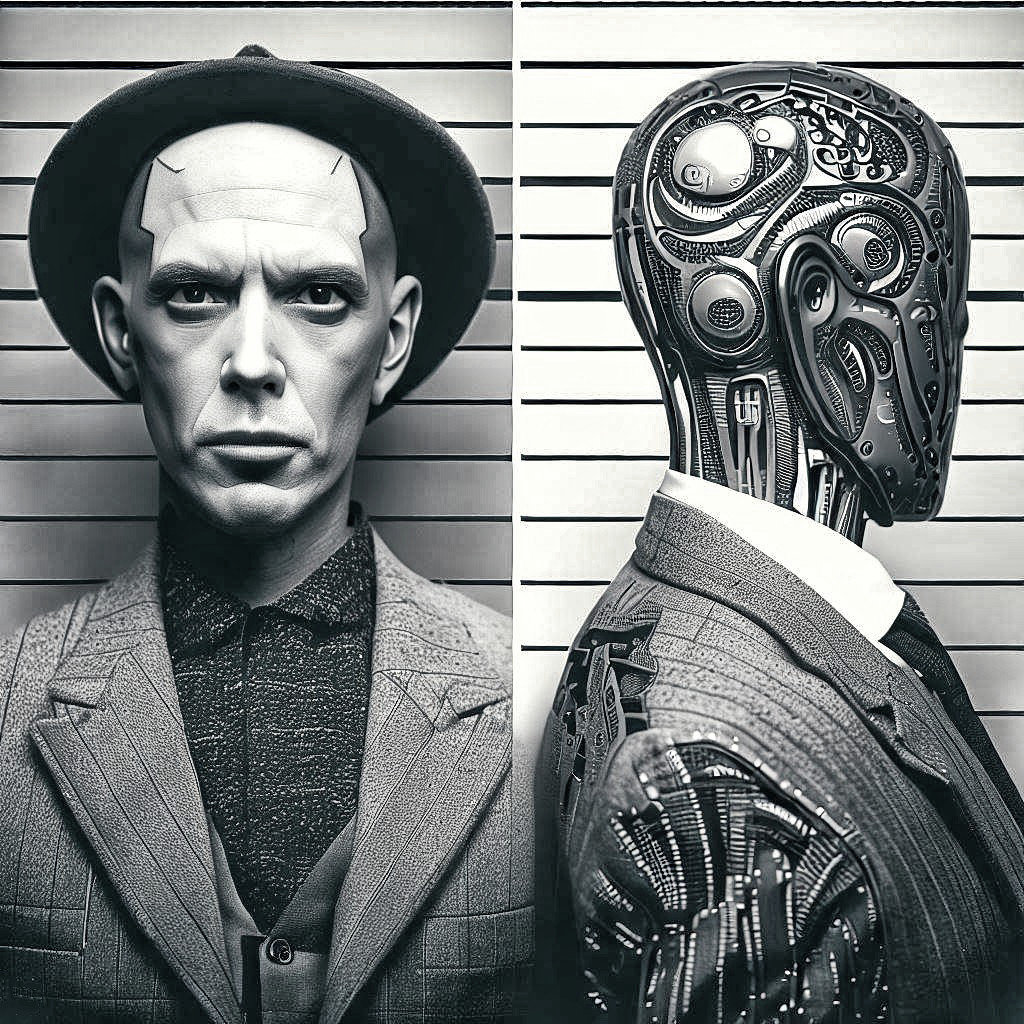
Recent breakthroughs in artificial intelligence (AI) have been driven by substantial increases in model scale enabled by computational advances. However, the vast data requirements of large neural network models raise critical questions around copyright compliance and attribution norms. In this paper, we analyze the copyright risks emerging from current AI training paradigms and present recommendations for responsible practice. AI Models Require Massive Training Data Most leading AI systems employ a transfer learning technique for model development. Models such as DALL-E 2, GPT-3, and Stable Diffusion are first pre-trained on large corpora of text, images, audio, video and other data scraped from publicly available sources. For instance, GPT-3 was trained on hundreds of billions of text tokens from books, Wikipedia articles, and webpages. Unsupervised pre-training objectives teach the models to encode generalized data representations across modalities...